The data life cycle is a framework that outlines the stages that data goes through from its initial creation or capture to its eventual deletion or archival. Here are the typical steps in the data life cycle:
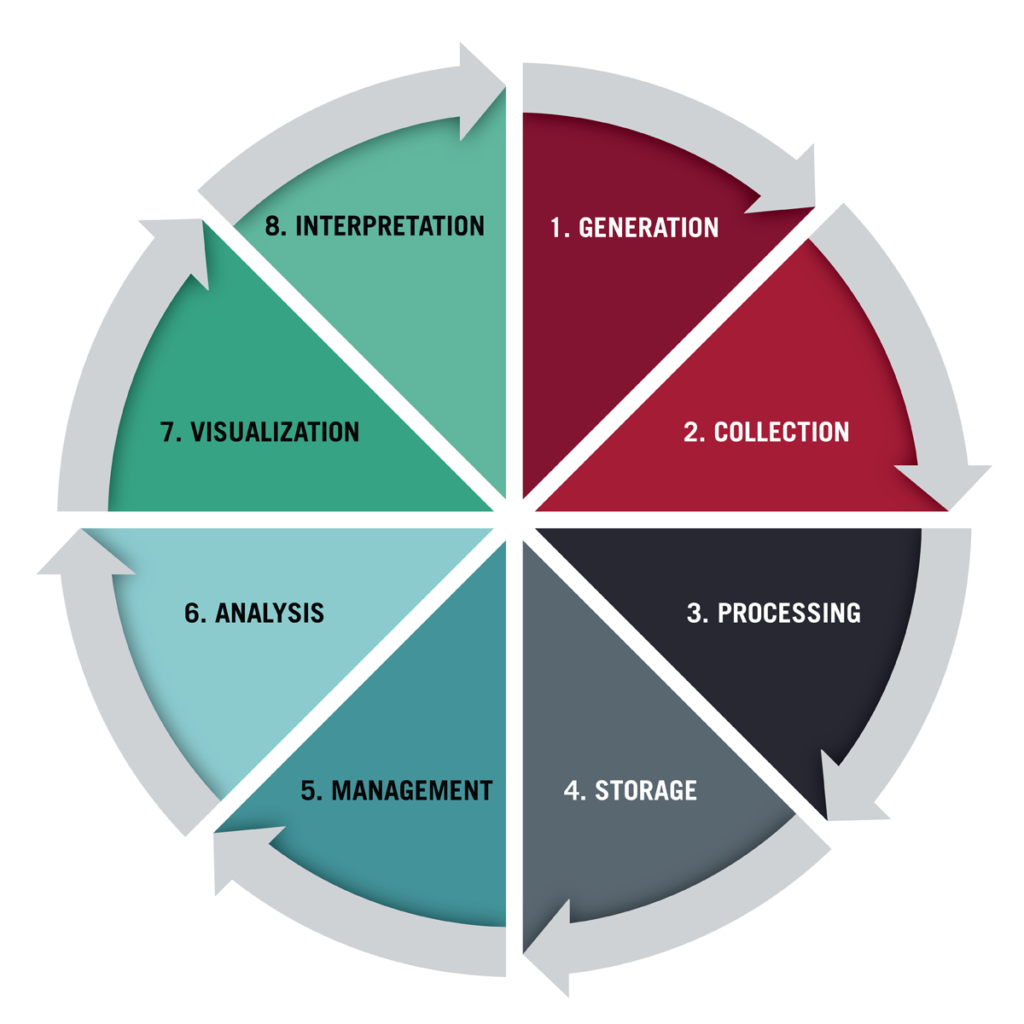
- Data Generation/Capture:
- This is the initial stage where data is created or captured. It can come from various sources such as sensors, user input, transactions, or any other form of data generation.
2. Data Ingestion:
- Once data is generated, it needs to be collected and stored in a structured manner. This might involve processes like data extraction, transformation, and loading (ETL) for further processing.
3. Data Storage:
- After ingestion, data needs to be stored in a secure and accessible location. This could be in a database, data warehouse, or other types of storage systems.
4. Data Processing:
- This step involves manipulating, cleaning, and transforming the raw data into a format that is suitable for analysis. It may include tasks like data normalization, aggregation, and filtering.
5. Data Analysis:
- Once the data is prepared, it can be analysed to extract meaningful insights. This is where various statistical and machine learning techniques are applied to uncover patterns, trends, or relationships within the data.
6. Data Visualization and Reporting:
- The results of the analysis are often communicated through visualizations or reports. This step helps in presenting the insights in a format that is easy to understand and interpret.
7. Data Archiving/Retirement:
- Over time, certain data may become less relevant for current analyses but may still need to be retained for legal or compliance reasons. Archiving involves moving data to a long-term storage solution.
8. Data Deletion/Disposition:
- Eventually, there may come a point where data is no longer needed and can be safely deleted. This step is crucial to ensure that unnecessary data is not taking up resources and to maintain compliance with data protection regulations.
It’s worth noting that some variations of the data life cycle might include additional steps or break down these steps further depending on the specific needs and requirements of an organisation or project. Additionally, with the advent of big data technologies and advanced analytics, the processes involved in each step may become more complex and sophisticated.